Download PDF
Researchers at the NEI have developed a deep learning framework that can diagnose and quantify cataracts with a high level of accuracy.1
“We believe that this is the first robust attempt to perform fully automated detection and quantitative classification of all three types of age-related cataract by deep learning models,” said Tiarnán D.L. Keenan, MD, PhD.
The researchers set out to determine whether artificial intelligence (AI) performance “could approach or equal human specialist performance,” Dr. Keenan added. “In this case, we were delighted to see that, in most cases, AI performance was significantly superior.”
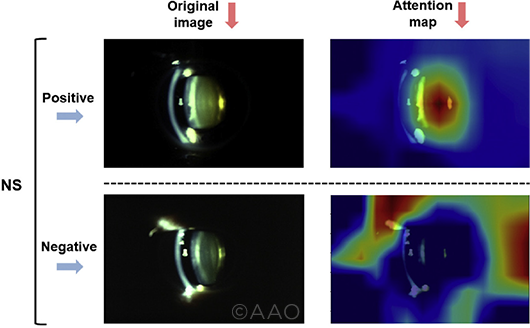 |
COMPARISON. In a case of severe nuclear sclerotic cataract (upper panel), the area of high signal corresponded to the location of the lens nucleus. In a mild case (lower panel), no areas of high signal were observed in the distribution of the lens nucleus.
|
Study design. For training, the researchers used 18,999 anterior segment images (from 6,333 triplets—i.e., slit-lamp, anterior retroillumination, and posterior retroillumination images) from 1,137 eyes. All images were from the Age-Related Eye Disease Study.
For validation, the researchers compared the performance of their AI models to that achieved by their 38 human participants. Of these, 14 were ophthalmologists (10 at attending level and four at fellowship level), and the remaining 24 were medical students with no experience in grading images.
Study results. The deep learning models achieved automated and quantitative classification of cataract severity with a high level of accuracy for nuclear, cortical, and posterior subcapsular cataracts. The main outcome measures were the mean squared error (MSE) for each test set.
For the two most common types—the nuclear and cortical cataracts—the model’s accuracy was significantly superior to that achieved by both the practicing ophthalmologists and the students. For nuclear cataracts, the mean MSE for the deep learning platform, ophthalmologists, and students was .23, .98, and 1.24, respectively. For cortical cataracts, these mean MSE values were 53.5, 134.9, and 422, respectively. For the less common posterior subcapsular cataracts, the model’s accuracy was similar to that achieved by the practicing clinicians (mean MSE, 171.9 vs. 176.8, respectively).
A surprise. On one hand, it’s no longer surprising that AI models approach, or even surpass, human specialists, Dr. Keenan said. “However, for nuclear sclerosis, the most common type of age-related cataract, we were surprised by the degree to which the AI models outperformed ophthalmologists, even those who are cataract specialists.”
Broad applications. Automated approaches are ideal for cataracts, which can be diagnosed and classified purely on anatomical appearance, the researchers noted. Their model is now ready for the research setting, where it is expected to be especially well-suited for the quantitative grading required in interventional clinical trials and epidemiological studies.
If approved by the FDA, such models could do everything from cataract screening in primary care to assisting surgical planning. The approach also lends itself to telemedicine, where it may enhance access to care, particularly in areas with few ophthalmologists.
Looking ahead, Dr. Keenan noted that “many single-domain tasks [in cataract care] will be well suited to AI, such as quantitative cataract classification, IOL calculation, and surgical risk prediction. Other tasks will remain best suited to humans, who can interpret complex information from diverse domains.”
At present, the models and associated code are available for research use only and may be found at https://github.com/ncbi-nlp/DeepSeeNet.
—Miriam Karmel
___________________________
1 Keenan TDL et al. Ophthalmology. Published online Jan. 3, 2022.
___________________________
Relevant financial disclosures: Dr. Keenan—None.
For full disclosures and the disclosure key, see below.
Full Financial Disclosures
Dr. Keenan NEI: E.
Dr. Seigfried NEI: S.
Dr. Margolin None.
Disclosure Category
|
Code
|
Description
|
Consultant/Advisor |
C |
Consultant fee, paid advisory boards, or fees for attending a meeting. |
Employee |
E |
Employed by a commercial company. |
Speakers bureau |
L |
Lecture fees or honoraria, travel fees or reimbursements when speaking at the invitation of a commercial company. |
Equity owner |
O |
Equity ownership/stock options in publicly or privately traded firms, excluding mutual funds. |
Patents/Royalty |
P |
Patents and/or royalties for intellectual property. |
Grant support |
S |
Grant support or other financial support to the investigator from all sources, including research support from government agencies (e.g., NIH), foundations, device manufacturers, and/or pharmaceutical companies. |
|
More from this month’s News in Review